AI Health
Friday Roundup
The AI Health Friday Roundup highlights the week’s news and publications related to artificial intelligence, data science, public health, and clinical research.
March 22, 2024
In this week’s Duke AI Health Friday Roundup: a framework for human labor in AI; the global health risks of air pollution; dermatology database seeks to overcome skin color bias in previous datasets; using generative AI for science communication; LLMs being used to generate peer reviews; the effects of digital redlining; AI-generated images used in engagement farming and scams; predicting underlying text from ground-truth embeddings; much more:
AI, STATISTICS & DATA SCIENCE
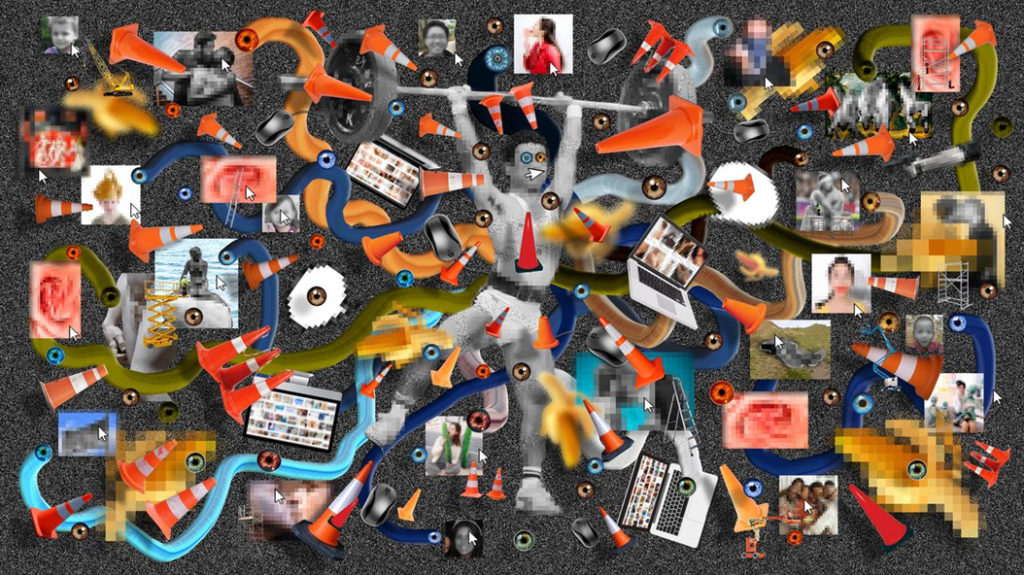
- “AI data work is conducted and managed across different employment structures and spatial distributions, which are also shaped by the specific histories and cultures of the geographic locations in which the work is performed. However, across all typologies, concerns about fairness and labour exploitation have been identified: from the low ratings of crowdsourced platforms to the recent media attention on Sama regarding labour exploitation and union busting…” An article by Muldoon and colleagues, published in the journal Big Data and Society, presents a schema for discussing the various kinds of human labor that underpin the functioning of artificial intelligence applications.
- “Our goal is now clear: we want to build a system that can take a ground-truth embedding, a hypothesis text sequence, and the hypothesis position in embedding space, and predict the true text sequence. We think of this as a type of ‘learned optimization’ where we’re taking steps in embedding space in the form of discrete sequences. This is the essence of our method, which we call vec2text….After working through some details and training the model, this process works extremely well!” At the Gradient, Jack Morris explores text embeddings, retrieval-augmented generation, and vector databases, and asks whether embedded vectors offer secure storage of information, or if those vectors can be inverted to yield the input text.
- “….dermatology conditions are diverse in their appearance and severity and manifest differently across skin tones. Yet, existing dermatology image datasets often lack representation of everyday conditions …and skew towards lighter skin tones. Furthermore, race and ethnicity information is frequently missing, hindering our ability to assess disparities or create solutions….To address these limitations, we are releasing the Skin Condition Image Network (SCIN) dataset in collaboration with physicians at Stanford Medicine.” As reported by Pooja Rao at the Google Research blog, a joint project by Stanford and Google Research aims to rectify a long-standing shortcoming in dermatology image databases.
- “In this study, we sought to discover what physicians and patients expect from digital agents (functional requirements) and how this functionality should be provided (nonfunctional requirements). A user-centric perspective is essential for guiding the development of digital agents because it prepares physicians for changes in their consultation methods and allows patients to understand what the new technology can offer.” A study published in JMIR Human Factors by Färber and colleagues reports findings from a series of interviews designed to elicit the attributes both physicians and patients would consider important for digital agents embedded in healthcare.
- “Our results suggest that between 6.5% and 16.9% of text submitted as peer reviews to these conferences could have been substantially modified by LLMs, i.e. beyond spell-checking or minor writing updates. The circumstances in which generated text occurs offer insight into user behavior: the estimated fraction of LLM-generated text is higher in reviews which report lower confidence, were submitted close to the deadline, and from reviewers who are less likely to respond to author rebuttals. We also observe corpus-level trends in generated text which may be too subtle to detect at the individual level, and discuss the implications of such trends on peer review.” A preprint paper by Liang and colleagues, available from arXiv, suggests that a noticeable percentage of peer reviews created for a series of AI conferences leaned substantially on the use of LLMs to generate the review text.
- “In both the JAMA Clinical Challenge and the NEJM Image Challenge databases, GPT-4V demonstrated significantly better accuracy than its unimodal predecessors, GPT-4 and GPT-3.5, and Gemini Pro, as well as the open-source models Llama 2 and Med42, confirming that GPT-4V can interpret medical images even without dedicated fine-tuning. Although the findings are promising, caution is warranted because these were curated vignettes from medical journals and do not fully represent the medical decision-making skills required in clinical practice…” A research letter published in JAMA by Han and colleagues describes an experiment that probed the ability of several large language models to respond correctly to a series of questions based on clinical vignettes.
BASIC SCIENCE, CLINICAL RESEARCH & PUBLIC HEALTH

- “The few oases of clean air that meet World Health Organization guidelines are mostly islands, as well as Australia and the northern European countries of Finland and Estonia. Of the non-achievers, where the vast majority of the human population lives, the countries with the worst air quality were mostly in Asia and Africa.” The New York Times’ Delger Erdenesanaa reports on the global pervasiveness of air pollution that exceeds health standards set by the World Health Organization.
- “Digital redlining refers to discriminatory disinvestment in broadband infrastructure and originates from historical redlining—a policy through which the US government intentionally segregated neighborhoods by excluding predominantly Black communities from homeownership and lending programs, leading to systemic economic and social disinvestment in these communities over time. Defined as racialized disparities in access to technology infrastructure, digital redlining drives and widens existing disparities in access to health care, education, employment, and social services for historically marginalized ethnic and racial groups…” A viewpoint article published in JAMA by Wang and colleagues examines the phenomenon of “digital redlining” and its deleterious impact on public health.
- “Evolutionary stasis, a phenomenon in which a lineage generates little phenotypic or species diversity over time, may explain why some branches on the Tree of Life are much less species rich and morphologically disparate than others. However, whether molecular rates of evolution are slower in living fossil lineages has not yet been confidently established. Here, using a sample of 1,105 exons, we show that several classic living fossil lineages, among them gars (Lepisosteidae) and sturgeons and paddlefishes (Acipenseriformes), possess exceptionally low genomic substitution rates.” A research article published in Evolution by Brownstein and colleagues examines a number of “living fossils” – species that undergo little evolutionary change over long intervals of time – and finds that they have distinctive genetic differences.
COMMUNICATION, Health Equity & Policy
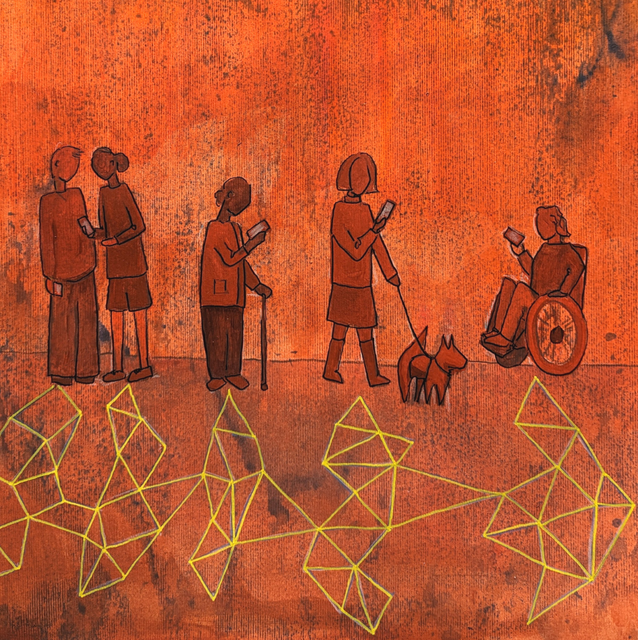
- “The companies with the most powerful AI models, such as GPT-4 and Gemini, will face more onerous requirements, such as having to perform model evaluations and risk-assessments and mitigations, ensure cybersecurity protection, and report any incidents where the AI system failed. Companies that fail to comply will face huge fines or their products could be banned from the EU.” At MIT Technology Review, Melissa Heikkila provides a quick rundown of the European Union’s AI Act, which is now law in the EU following a long period of discussion and rounds of approval.
- “Evidently, developing generative AI goes beyond trustworthy AI; trustworthy science communicators are also part of the technological ecosystem. Can the use of generative AI tools by science communicators measure up to public expectations of trustworthiness? This is an important question to ponder as the interaction between science communicators and the next generation of generative AI co-evolves.” An article at Nature Human Behavior taps expert advice on how generative AI can be used for science communication.
- “Reparative description focuses on remediating or contextualizing potentially outdated or harmful language used in descriptive practices, ensuring accuracy and inclusivity…” The Digital Public Library of America’s Metadata Working Group has announced a series of workshops on the practice of reparative description for archival materials.
- “…that capacity to produce captivating, novel, and immersive imagery, cheaply and instantly, and to immediately double down on wins that generate significant engagement, is also what makes the technology appealing to spammers and scammers. These innovative actors, seemingly motivated primarily by profit or clout (not ideology) have been using AI-generated images to gain viral traction on Facebook since AI image-generation tools became readily available.” A blog entry at the Stanford Internet Observatory describes findings from a recent preprint that traces the sudden proliferation of surreal AI-generated art on Facebook to the scam-infested social media attention subeconomy.