AI Health
Friday Roundup
The AI Health Friday Roundup highlights the week’s news and publications related to artificial intelligence, data science, public health, and clinical research.
April 12, 2024
In this week’s Duke AI Health Friday Roundup: new pretraining approach allows LLMs to cite sources; Light Collective releases draft guidance on AI rights for patients; NYC government chatbot delivers dubious advice; study evaluates precision medicine approach in pediatric cancers; weighing up AI X-risk; new analyses cast doubt on DIANA fMRI technique; counting the full data costs of zero-shot learning for multimodal generative AIs; much more:
AI, STATISTICS & DATA SCIENCE
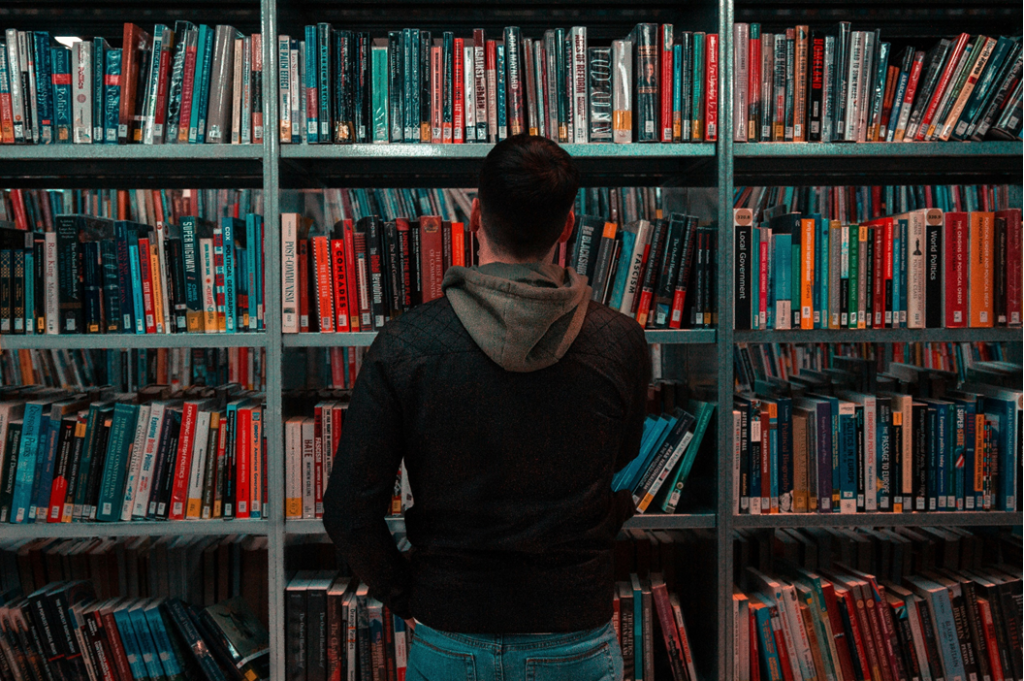
- “We investigate the problem of intrinsic source citation, where LLMs are required to cite the pretraining source supporting a generated response. Intrinsic source citation can enhance LLM transparency, interpretability, and verifiability. To give LLMs such ability, we explore source-aware training—a post pretraining recipe that involves (i) training the LLM to associate unique source document identifiers with the knowledge in each document, followed by (ii) an instruction-tuning to teach the LLM to cite a supporting pretraining source when prompted.” In a preprint available on arXiv, Khalifa and colleagues present a new approach to large language model pretraining that allows the LLM to cite the sources of the information it provides in response to a prompt.
- “An analysis of the annotations reveals that most unfaithful claims relate to events and character states, and they generally require indirect reasoning over the narrative to invalidate. While LLM-based auto-raters have proven reliable for factuality and coherence in other settings, we implement several LLM raters of faithfulness and find that none correlates strongly with human annotations, especially with regard to detecting unfaithful claims. Our experiments suggest that detecting unfaithful claims is an important future direction not only for summarization evaluation but also as a testbed for long-context understanding.” A research article by Kim and colleagues, available as a preprint from arXiv, evaluates the performance of several large language models tasked with providing concise summaries of book-length fiction.
- “Our novel AI-based system utilizing ISL [inverse supervised learning] can accurately and broadly detect disorders without requiring disorder-containing data. This system not only outperforms previous AI-based systems in terms of disorder detection but also provides visually understandable clues, enhancing its clinical utility.” A research paper by He and colleagues published in NEJM AI presents findings from a study of AI image recognition that inverts the usual approach – instead of attempting to train the model to identify anomalies, the system was trained solely on brain images without any disorders.
- “We consistently find that, far from exhibiting “zero-shot” generalization, multimodal models require exponentially more data to achieve linear improvements in downstream “zero-shot” performance, following a sample inefficient log-linear scaling trend. This trend persists even when controlling for sample-level similarity between pretraining and downstream datasets, and testing on purely synthetic data distributions. Furthermore, upon benchmarking models on long-tailed data sampled based on our analysis, we demonstrate that multimodal models across the board perform poorly.” A study by Undandarao and colleagues, available as a preprint from arXiv, presents findings that call into question whether the performance of “zero-shot” learning approaches to multimodal generative AIs is dependent on the frequency with which a particular concept appears in the training data.
BASIC SCIENCE, CLINICAL RESEARCH & PUBLIC HEALTH

- “Some public health workers worry that AI will replace them. To the contrary, a renewed commitment to the health, retention, and professional development of the public health workforce—a precious and diminishing resource—is inherent in the project to develop human-AI centaurs in public health. An AI tool is only as reliable as its training data set and the sophistication of its user. AI tools may have the capacity to quickly summarize data or generate content, but it falls on a human subject-matter expert to appropriately prompt the machine, to assess the credibility of the output, and to respond in a way that produces results in the real world.” An essay by Cullen and colleagues appearing in Health Affairs Forefront makes a case for equipping population health workers with the tools of AI and data science.
- “We demonstrate the feasibility of returning a combination of drug sensitivity profiles and molecular data (FPM) to clinicians to inform subsequent treatment recommendations for pediatric patients with relapsed or refractory cancers. This prospective study highlights the use of FPM data to inform the next line of therapy for children who have exhausted standard-of-care options.” A study published this week in Nature Medicine reports findings from a nonrandomized observational study that applied precision medicine tools to optimize treatment for pediatric cancer patients with recurrent or refractory cancers.
- “…the latest Science Advances papers have cast doubt on the original findings. It’s clear that the signals DIANA detects are ‘not necessarily related to neural signal’, says Shella Keilholz, an MRI physicist and neuroscientist at Emory University in Atlanta, Georgia. Although, she says, it’s possible that brain activity contributed to the detected signals….Neuroscientists will continue to explore the cause of the conflicting results.” A news article by Nature’s McKenzie Prillaman reports on recently published findings that cast doubt on an fMRI brain imaging technique known as direct imaging of neuronal activity (DIANA).
COMMUNICATION, Health Equity & Policy

- “In the current landscape, health systems and technology developers are fervently embracing artificial intelligence AI tools to assist clinicians in their roles, and facilitate various other processes in the healthcare system. However, amid this haste, there persists a significant oversight—the exclusion of patient perspectives in the design and governance of AI solutions. The omission of patient representation repeats history we do not want to see: a power dynamic where those with the most influence in healthcare at best tokenize and at worst ignore the voices of those they serve.” Patient advocacy organization The Light Collective has released a draft guidance on patient rights with regard to the use of AI in healthcare.
- “The bot said it was fine to take workers’ tips (wrong, although they sometimes can count tips toward minimum wage requirements) and that there were no regulations on informing staff about scheduling changes (also wrong). It didn’t do better with more specific industries, suggesting it was OK to conceal funeral service prices, for example, which the Federal Trade Commission has outlawed. Similar errors appeared when the questions were asked in other languages, The Markup found.” At the Markup, Colin Lecher reports on problematic advice being dispensed by New York City government’s public-facing information chatbot.
- “Many longer-term risks of AI, though potentially dire, still seem very hypothetical — we have yet to see an AI so clever it can outwit a human and take over a military installation or launch a war. But there can be no doubt that AI is already being used to take advantage of Americans literally every day, and that we have too little in place to defend against these growing threats.” In an lengthy and detailed opinion article for Politico Magazine, Gary Marcus enumerates a number of threats posed by AI – and recommends approaches for mitigating them.
- “Before determining what moral weight to assign AI X-Risk, consider non-AI X-Risks. For example, an increasing number of bacteria, parasites, viruses and fungi with antimicrobial resistance could threaten human health and life; the use of nuclear, chemical, biological or radiological weapons could end the lives of millions or make large parts of the planet uninhabitable; extreme weather events caused by anthropogenic climate change could endanger the lives of many people, trigger food shortages and famine, and annihilate entire communities. Discussion of these non-AI X-Risks is conspicuously absent from most discussions of AI X-Risk.” An article by Jecker and colleagues appearing in the Journal of Medical Ethics attempts to weigh up the actual threat posed by supposedly existential perils inherent in the advance of AI (known as “X-risk.”