AI Health
Friday Roundup
The AI Health Friday Roundup highlights the week’s news and publications related to artificial intelligence, data science, public health, and clinical research.
May 24, 2024
In this week’s Duke AI Health Friday Roundup: an ML-enabled intervention provides nudges for hard medical conversations; informational “inoculation” for misinformation; understanding what LLMs can and can’t do; power dynamics affect medical care; assessing the impacts of race-based adjustments for lung function; quantum internet marks another milestone; links between race, environmental pollution, and Alzheimer disease; much more:
AI, STATISTICS & DATA SCIENCE
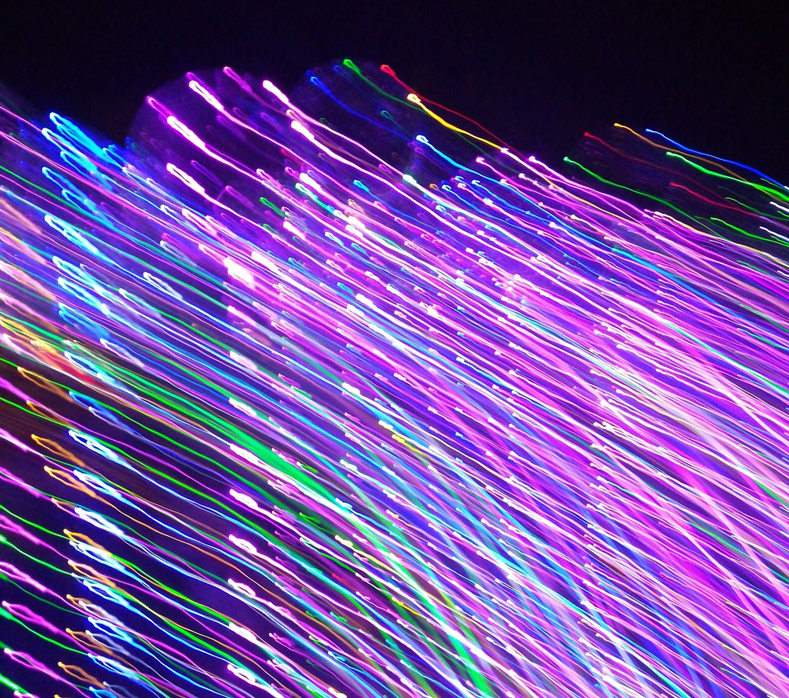
- “Three separate research groups have demonstrated quantum entanglement — in which two or more objects are linked so that they contain the same information even if they are far apart — over several kilometres of existing optical fibres in real urban areas. The feat is a key step towards a future quantum internet, a network that could allow information to be exchanged while encoded in quantum states.” In a news article for Nature, Davide Castelvecchi reports on the attainment of an important milestone in the development of a quantum internet.
- “A machine learning–based, behaviorally informed intervention to prompt SICs [serious illness conversations] led to end-of-life savings among patients with cancer, driven by decreased systemic therapy and outpatient spending.” A recent publication in NEJM AI by Patel and colleagues describes a machine-learning-enabled behavior economics intervention designed to “nudge” clinicians and patients to engage in conversations about serious illnesses.
- “So, does GPT-4 genuinely know and understand these concepts? There is heated debate over understanding in LLMs, including the very definition of what it means to “understand.” Regardless of where you stand on this debate, we need to look beyond the headline-grabbing test results and reconsider how we evaluate and promote LLMs.” In an editorial for Radiology, Woojin Kim stresses the need for “humility and caution” when evaluating the merits of AI-based applications in medicine.
- “Starting in the 19th century, [countries] began conducting population censuses, creating civil registers, and establishing statistical agencies. In the later 20th century, they started setting up population registers and using register-based censuses. Thanks to these efforts, these countries better understand where people live, what jobs they have, who was born, and who has died. However, many countries still lack these institutions, which makes it challenging for them to direct projects and policies where they are most helpful.” An interactive chart published by Our World in Data looks at the growth of national-level statistics organizations over time.
- “Astronomers had already been using AI models for years, mainly to classify known objects such as supernovas in telescope data. This kind of image recognition will become increasingly vital when the Vera C. Rubin Observatory opens its eyes next year and the number of annual supernova detections quickly jumps from hundreds to millions. But the new wave of AI applications extends far beyond matching games. Algorithms have recently been optimized to perform ‘unsupervised clustering,’ in which they pick out patterns in data without being told what specifically to look for. This opens the doors for models pointing astronomers toward effects and relationships they aren’t currently aware of.” In an article for MIT Technology Review, Zack Savitsky describes the dawning of new age in astronomy as AI-powered data capabilities advance in tandem with larger, more sophisticated instruments.
- “In previous writings I talked about the difficulties in evaluating the capabilities of large language models. These models have excelled on many benchmarks, but we typically don’t know the extent to which the test items in a benchmark—or sufficiently similar items—appeared in the training data. Are these models understanding and reasoning in a general way, or are they doing what AI researcher Subbarao Kambhampati calls “approximate retrieval” — relying on patterns of text that are contained in the model’s training data?” In a Substack post at AI: A Guide for Thinking Humans, Melanie Mitchell walks readers through how to think about how large language models work – and how to interpret the ways they perform (or fail to perform) tasks.
BASIC SCIENCE, CLINICAL RESEARCH & PUBLIC HEALTH

- “Research linking social media use and adolescent mental health has produced mixed and inconsistent findings and little translational evidence, despite pressure to deliver concrete recommendations for families, schools and policymakers. At the same time, it is widely recognized that developmental changes in behaviour, cognition and neurobiology predispose adolescents to developing socio-emotional disorders…. we review mechanisms by which social media could amplify the developmental changes that increase adolescents’ mental health vulnerability.” In a review article published in Nature Reviews Psychology, Orben and colleagues survey what is currently known about how the use of social media interacts with adolescent development.
- “By comparing the results obtained with the use of race-stratified GLI-2012 equations with those obtained with race-neutral GLI-Global equations, our analyses showed that the choice of including or removing adjustment for race does not meaningfully change the discriminative accuracy of relevant clinical outcomes but reclassifies lung diseases, occupational eligibility, and disability compensation for millions. These findings underscore the extent of medical decision making that is at stake with the use of race-based equations and warrant thoughtful consideration of the trade-offs involved.” A research article by Diao and colleagues, published in the New England Journal of Medicine, examines the use of adjustments based on racial categories in evaluating lung function.
- “Medical professionals can help patients identify incorrect information and avoid accepting it as fact. Although it may seem unusual to address misinformation before a patient brings it up, this method can be effective in preventing the adoption of inaccurate information….Inoculating against common manipulation techniques can be useful in helping people understand why certain claims are unreliable and how they may be dangerous to their health.” An article appearing in JAMA Insights by van der Linden and Roozenbeek explores the strategy of informational “inoculation” to prevent the spread of medical misinformation.
- “The results add to a growing area of research exploring the connections between environmental factors and brain health, racial injustices, and aging, and suggests looking at a patient’s address may be just as important for care providers to consider as listening to their heart or ordering a brain scan.” A web article by Duke’s Dan Vahaba highlights recent research by investigators from Duke and Columbia that found associations between the degree of environmental pollution in neighborhoods and risk for developing Alzheimer disease among Black adults.
COMMUNICATION, Health Equity & Policy
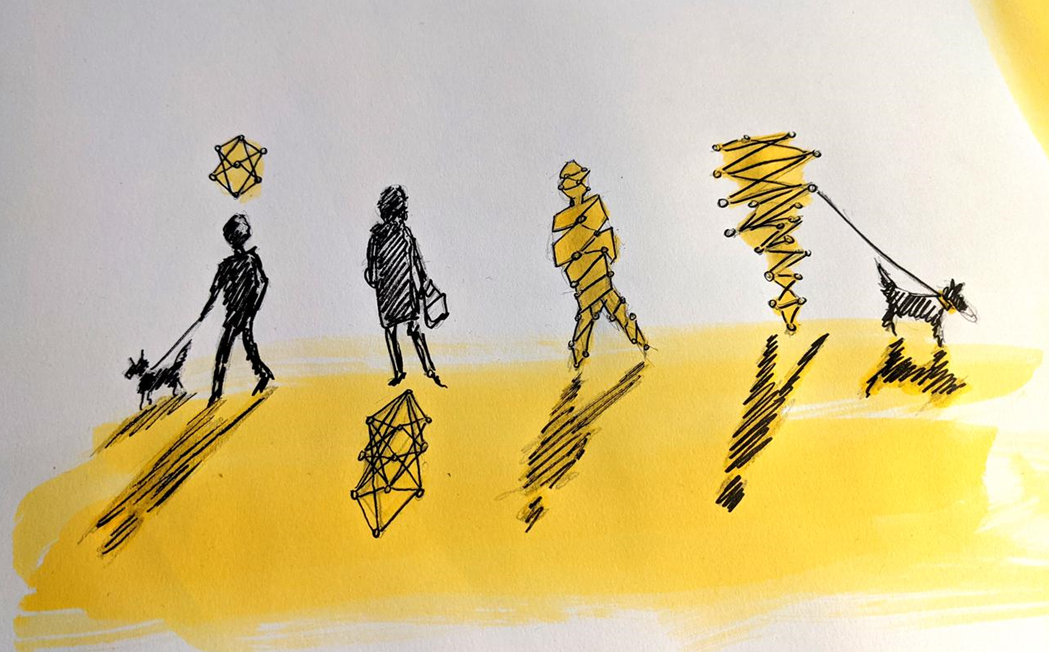
- “Technology is built by humans and controlled by humans, and we cannot talk about technology as an independent agent acting outside of human decisions and accountability….The integrity that Mann rightly envisions for AI cannot be understood as a property of a model, or of a software system into which a model is integrated. Such integrity can only come via the human choices made, and guardrails adhered to, by those developing and using these systems. This will require changed incentive structures, a massive shift toward democratic governance and decision making, and an understanding that those most likely to be harmed by AI systems are often not ‘users’ of the systems, but subjects of AI’s application ‘on them’ by those who have power over them…” The Innovator’s Jennifer L. Schenker interviews Signal president and AI Now Institute co-founder Meredith Whittaker.
- “Using 1.5 million quasi-random assignments in US military emergency departments, we examined how power differentials between doctor and patient (measured by using differences in military ranks) affect physician behavior. Our findings indicate that power confers nontrivial advantages: ‘High-power’ patients (who outrank their physician) receive more resources and have better outcomes than equivalently ranked ‘low-power’ patients. Patient promotions even increase physician effort.” An article published in Science by Schwab and Singh examines the workings of power dynamics in patient care in military settings.
- “As clinical psychologist Simon Baron-Cohen has shown, there’s more neurodiversity in science compared to other fields because many scientists are the systematizing thinkers that he calls “pattern seekers,” a common trait of autism. Some neurodivergent people are meticulously observant and are able to connect seemingly disparate concepts—assets in the world of science. This should make science a comfortable place to call home, yet not everyone feels so included. This must improve.” An editorial in Science by Holden Thorp explains why the enterprise of science needs to make room for neurodiversity.