AI Health
Friday Roundup
The AI Health Friday Roundup highlights the week’s news and publications related to artificial intelligence, data science, public health, and clinical research.
October 18, 2024
In this Friday’s Duke AI Health Friday Roundup: framework for aligning AI with the needs of clinicians, patients; even small amounts of synthetic data tied to model collapse; FDA perspective on AI regulations; evaluating satisfaction with AI responses to patient questions; exposure to COVID during pregnancy not associated with later effects on infants; probing the limits of LLM reasoning; revisiting the early days of peer review; tracking scholarly content licensing for AI training; much more:
AI, STATISTICS & DATA SCIENCE
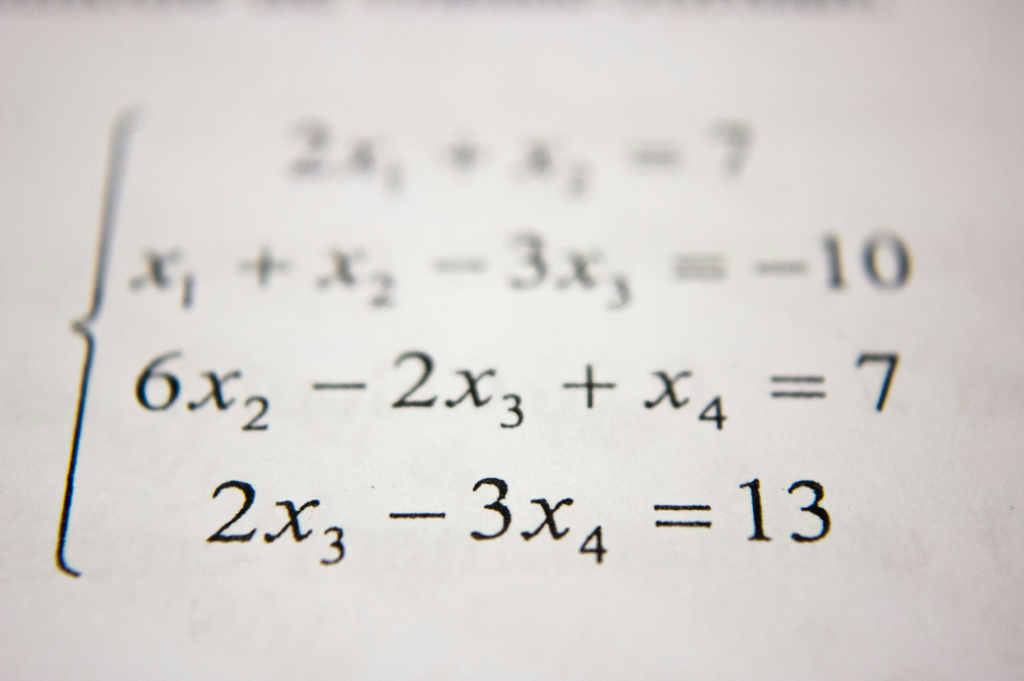
- “…LLMs exhibit noticeable variance when responding to different instantiations of the same question. Specifically, the performance of all models declines when only the numerical values in the question are altered in the GSM-Symbolic benchmark. Furthermore, we investigate the fragility of mathematical reasoning in these models and demonstrate that their performance significantly deteriorates as the number of clauses in a question increases. We hypothesize that this decline is due to the fact that current LLMs are not capable of genuine logical reasoning; instead, they attempt to replicate the reasoning steps observed in their training data.” A preprint by researchers from Apple explores limitations of “reasoning,” especially mathematical reasoning, in current large language models.
- “I do think, however, that we currently lack a lot of the standards when it comes to developing predictive AI. One example is that unlike regular medical technology, when we develop AI systems, they are sensitive to the distribution in which they’re deployed. So it’s not enough to have a one-size-fits all tool that is developed once and then used in hospitals over time and across the country. What we really need to have is domain-specific interventions, so a tool that’s fine-tuned to work well within a specific hospital system or even a specific hospital.” A STAT News interview with Sayash Kapoor delves into both the real promise and counterproductive hype surrounding generative AI in medicine.
- “Our results show that even the smallest fraction of synthetic data (e.g., as little as 1% of the total training dataset) can still lead to model collapse: larger and larger training sets do not enhance performance. We further investigate whether increasing model size, an approach aligned with current trends in training large language models, exacerbates or mitigates model collapse. In a simplified regime where neural networks are approximated via random projections of tunable size, we both theoretically and empirically show that larger models can amplify model collapse. Interestingly, our theory also indicates that, beyond the interpolation threshold (which can be extremely high for very large datasets), larger models may mitigate the collapse, although they do not entirely prevent it.” A preprint by Dohmatob and colleagues, available from arXiv, demonstrates modes in which large language models undergo the phenomenon of model collapse when even relatively small proportions of synthetic data are included in the model’s training.
- “Satisfaction was consistently higher with AI-generated responses than with clinicians overall and by specialty. However, satisfaction was not necessarily concordant with the clinician-determined information quality and empathy. For example, satisfaction was highest with AI responses to cardiology questions while information quality and empathy were highest in endocrinology questions. Interestingly, clinicians’ response length was associated with satisfaction while AI’s response length was not.” A research letter, published in JAMA Network Open by Kim and colleagues, explores the question of patient satisfaction vs physician-assessed quality regarding AI-generated responses to patients’ medical questions.
BASIC SCIENCE, CLINICAL RESEARCH & PUBLIC HEALTH
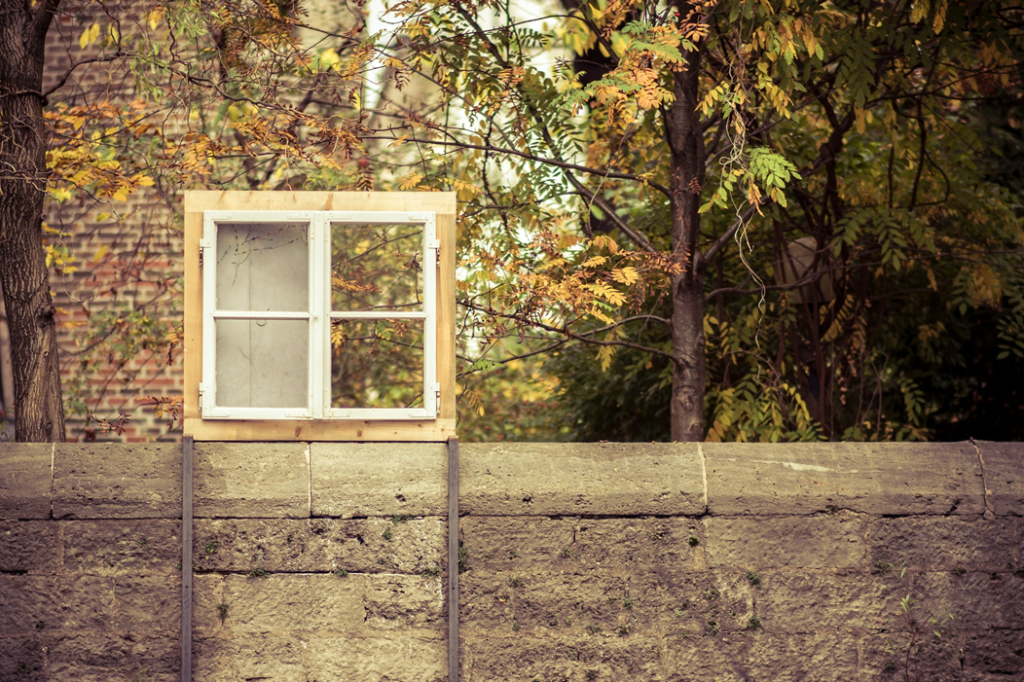
- “…this potential will not be realized without a refocusing of AI technology development toward a closer alignment with the health goals that clinicians and patients understand are required to ensure widespread adoption and maximal impact to improve human health. We need clearly articulated clinical indications, well-defined risk-based clinical testing processes and evidence generation, and continuous monitoring linked to these indications.” A viewpoint article published this week in JAMA by Patel, Balu, and Pencina lay out a framework for aligning the development and use of AI tools in a way that supports the needs of both clinicians and patients.
- Boyles’ Law and Brownian motion for tots, per Nature research highlights: “When the children were allowed to roam freely in the playground, their positions, when averaged out, followed statistical patterns similar to those of molecules in a gas (even if individual children did not move like molecules). But during the partially restricted classroom activities, the kids tended to form temporary clusters. This pattern resembles the liquid–vapour coexistence phase of water, in which freely moving individual gas molecules coexist with liquid droplets….Data about the orientation of each child’s body revealed arrangements similar to those of atoms in both magnetic and non-magnetic materials.”
- “…in utero exposure to maternal SARS-CoV-2 infection was not associated with abnormal neurodevelopmental screening scores of children through age 24 months. These findings are critical considering the novelty of the SARS-CoV-2 virus to the human species, the global scale of the initial COVID-19 outbreak, the now-endemic nature of the virus indicating ongoing relevance for pregnant individuals, the profound host immune response noted in many patients with COVID-19, and the accumulating evidence revealing sensitivity of the developing fetal brain to maternal immune activation.” A research article published in JAMA Network Open by Jaswa and colleagues examines whether a mother’s exposure to COVID while pregnant has downstream neurodevelopmental implications for children.
- “…delandistrogene moxeparvovec did not show a statistically significant difference compared to placebo in the primary endpoint at week 52. Key secondary endpoints and other functional endpoints numerically favored delandistrogene moxeparvovec in the overall population and age subgroups, although no statistical significance can be claimed.” A research paper published in Nature Medicine by Mendell and colleagues reports results from phase 3 trial of gene therapy for the treatment of Duchenne muscular dystrophy.
COMMUNICATION, Health Equity & Policy
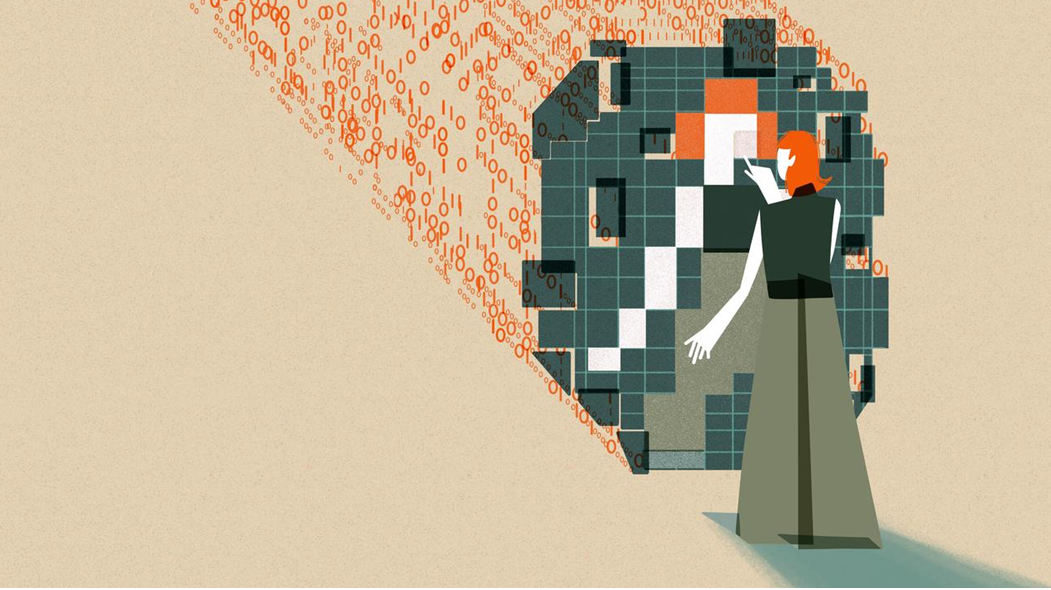
- “The basic idea behind these deals is to generate revenue for the publishing house in exchange for easy, reliable, and legal access to the content for the LLM. A number of companies are in the hunt for this content, including not only OpenAI and Google, but also Apple and more specialized providers. Investment has been pouring in as a result of the market’s spike in interest in artificial intelligence, and so striking deals now allows publishers to cash in before this investment dries up.” In a post for Scholarly Kitchen, Roger Schonfeld describes a new tracker developed to keep tabs on the state of play regarding the licensing of scholarly publications for use in training large language models.
- “Many of the concerns surrounding open foundation models arise from the fact that once model weights are released, developers relinquish control over their downstream use. Even if developers attempt to restrict downstream use, such restrictions can be ignored by malicious users. By contrast, in the face of malicious use, developers of closed foundation models can restrict access to them. It should be stressed, however, that this categorical distinction may oversimplify the gradient of model release: Closed models are also susceptible to malicious use, given that current safeguards are circumventable.” A Science policy forum article by Bommasani and colleagues lays out elements for governance framework for open foundation model AIs.
- “Historic advances in AI applied to biomedicine and health care must be matched by continuous complementary efforts to better understand how AI performs in the settings in which it is deployed. This will entail a comprehensive approach reaching far beyond the FDA, spanning the consumer and health care ecosystems to keep pace with accelerating technical progress.” A JAMA special communication by Warraich, Tazbaz, and Califf lays out FDA thinking regarding regulation of AI tools for health and healthcare applications.
- “The notion that peer review should preserve the quality of the scientific record is a modern viewpoint. The Royal Society archive demonstrates another, less noble, reason to use referees: to help journals control costs…In a 1943 review by Haldane of a paper on the fecundity of Drosophila flies, the reviewer suggested cutting figures and tables, some of the discussion and, bizarrely, a short eulogy of a fly biologist. The authors followed the suggestions in full.” Nature’s David Adams reports on the Royal Society’s release of a trove of materials from the dawn age of peer review.