Building Better Guardrails for Algorithmic Medicine
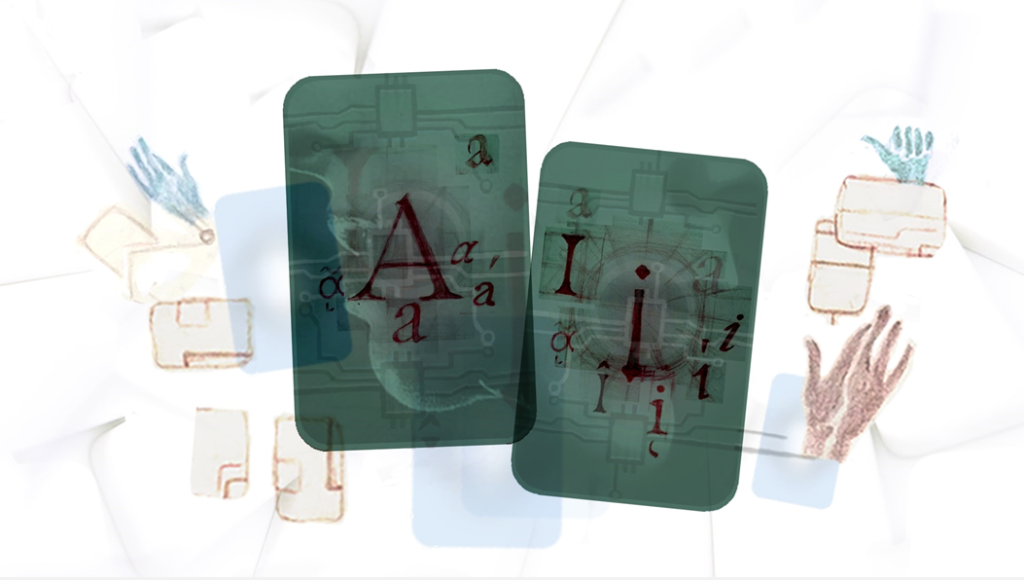
By Jonathan McCall, MS
Recent years have seen growing interest in the use of artificial intelligence tools for healthcare applications, including diagnosis, risk prediction, clinical decision support, and resource management. Capable of finding hidden patterns within the enormous amounts of data that reside in patient electronic health records (EHRs) and administrative databases, these algorithmic tools are diffusing across the world of patient care. Often, health AI applications are accompanied by assurances of their potential for making medical practice better, safer, and fairer.
The reality, however, has turned out to be more complex.
“AI for healthcare is going through a sort of ‘Wild West’ period,” says Duke AI Health Director and Vice Dean for Data Science Michael Pencina, PhD. “Many hospitals and health systems have gone off on their own and built or purchased systems and are running them on their own data, sometimes with little oversight.”
Enthusiasm for applying AI tools to some of healthcare’s most vexing problems is helping drive the adoption of technologies that, until relatively recently, had not undergone the kinds of rigorous scrutiny routinely applied to drugs and medical devices. And for a growing number of practitioners and health systems, worries are mounting that some of these tools – many of which are designed as inscrutable “black box” systems – might not be working as they should.

These concerns recently came to wider public awareness when researchers at the University of Michigan investigated the performance of an algorithmic tool designed to alert clinical staff to the possible presence of sepsis, a serious medical condition. The researchers realized that the tool was performing worse than expected in the real world of patient care, flagging nonexistent cases of sepsis and missing actual ones. Prompted by this and other examples of erring algorithms, experts have grown increasingly concerned about the potential for malfunctioning AI tools to compromise quality and safety or to reinforce existing inequities.
The Need for Algorithmic Oversight
Although there are numerous reasons why the performance of an AI system can decline, many can be traced back to decisions made during the system’s design phase, and most critically, to differences between the data used to train the system versus the kinds of data the system encounters once it is applied in the clinic. For this reason, algorithmic tools must be carefully validated during their creation, closely scrutinized throughout their lifecycles, and continuously monitored after deployment.
“It’s become quite clear that the current situation has to change,” says Pencina, who notes that a groundswell of interest in establishing shared best practices and common approaches to regulating AI healthcare tools has been building for some time.
Indeed, at Duke that concern has translated into the creation of a system of governance and oversight for AI tools. Dubbed the Algorithm-Based Clinical Decision Support (ABCDS) Oversight Committee and co-chaired by Pencina and by Duke Health Chief Health Information Officer Eric Poon, MD, ABCDS Oversight represents a collaboration spanning both Duke University and the Duke University Health System.
“ABCDS Oversight allows us to ensure that quality and equity are built into all of the algorithmic tools developed or used at Duke Health,” says Poon. “We’ve evolved an approach that convenes experts from all relevant domains – AI, clinical specialty practice, IT, regulatory, and more. Those experts offer input and guidance at the earliest stages of model development. The idea is to ensure that tools demonstrate impact on the key goal of improving how we deliver patient care.”

Contributing to the Bigger Picture
Given Duke’s early adoption of rigorous approaches to algorithmic oversight, it’s unsurprising to see it assuming a role in a new national consortium, the Coalition for Health AI (CHAI), that is convening experts from academia, industry, and regulatory agencies to thresh out urgent issues related to the ethical and equitable use of AI tools for health and healthcare. Chief among these are the need to harmonize multiple competing recommendations for reporting standards, and ensuring that fairness and equity are built into health AI systems from the ground up.
These are critically important considerations, because end users, patients, and consumers might not trust AI systems if shared standards and guidelines are not clearly understood – or worse, are missing altogether. Transparency and trustworthiness are key to ensuring that health AI practices can be effectively applied to improve care for the under-served and under-represented patients and communities who are most impacted by inequity.
“It’s inspiring to see the AI and data science community come together to harmonize standards and reporting for high-quality, fair, and trustworthy health AI. To evaluate these AI systems and increase their credibility, experts from academic health systems, healthcare organizations, and industry partners are also connecting the dots between data scientists, policymakers, and the broader community of those developing and using AI in healthcare,” says ABCDS Oversight Director Nicoleta J. Economou-Zavlanos, PhD, who is also co-leading CHAI’s efforts. “We’re also benefiting from the insights of those who are directly impacted by these AI technologies. CHAI is committed to giving all stakeholders a seat at the table and a voice in the debate over how to govern these incredibly powerful tools.”
The Coalition for Health AI seeks to create “guidelines and guardrails” that will enable the development of health AI systems that are “credible, fair, and transparent.” A first step toward this goal is a framework, arrived at through discussion and consensus among partners and stakeholders, including end users and patients. The framework will define key precepts, standards, and criteria that will be used by those who develop, deploy, and use AI systems in healthcare to monitor and evaluate their performance throughout a given application’s lifecycle.

One of CHAI’s immediate goals is to set standards that will result in health AI systems that can drive high-quality care, increase credibility among users, and meet healthcare needs. Following an initial announcement of the group’s formation and intent, CHAI has spent the last few months convening a series of virtual meetings, focused on the themes of testability, usability, safety, transparency, reliability and monitoring to explore different areas of interest in health AI through illustrative use cases.
These meetings culminated in a hybrid in-person/virtual meeting (with support from the Partnership on AI and funding from the Gordon and Betty Moore Foundation) that set the stage for the creation of a set of guidelines and recommendations. Each meeting has been accompanied by a “readout” paper capturing meeting presentations and discussions. Most recently, the Coalition has released a Draft Blueprint For Trustworthy AI Implementation Guidance and Assurance for Healthcare and is soliciting comments and feedback from the public.
“What’s really exciting about CHAI is that it represents an opportunity for stakeholders to convene and build consensus around a framework that will ensure that AI gets used in ways that are genuinely beneficial at all levels,” says Duke AI Health Associate Director Andrew Olson, MPP.
In a blog post recently shared on the CHAI website, co-chair Michael Pencina underscored the Coalition’s “strong commitment to making equity the cornerstone of the ethical framework we are trying to build for AI in healthcare.” Pencina further noted that the ability to engage meaningfully with all stakeholders affected by health AI was essential to fostering trust in such tools.
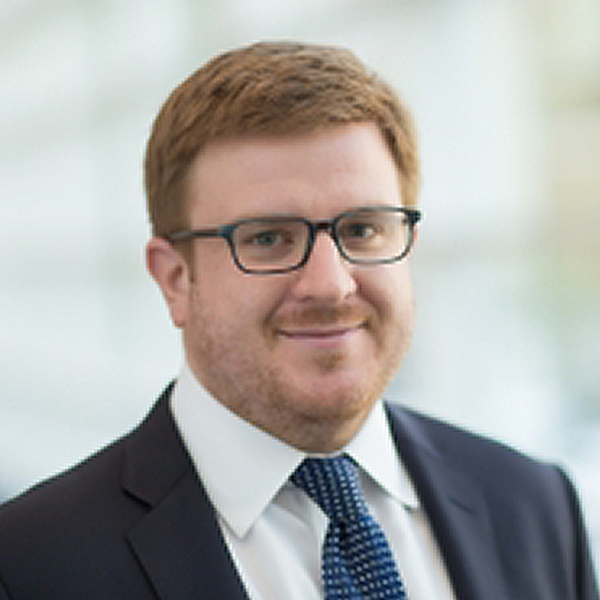
In addition to Duke AI Health, CHAI’s growing list of partners includes Stanford University, UC San Francisco, Johns Hopkins University, UC Berkeley, the Mayo Clinic, MITRE Health, Change Healthcare, Microsoft Corporation, SAS, and Google, among others. Observers from the US Food and Drug Administration, which exercises regulatory oversight of health AI applications that meet certain criteria, and from the National Institutes of Health and the Office of the National Coordinator for Health Information Technology, were also present at recent CHAI meetings.
As work by CHAI and its partners continues, complementary efforts are also underway at the federal level, with the FDA’s publication of a final guidance concerning clinical decision support software and a Blueprint for an AI Bill of Rights published by the White House Office of Science and Technology Policy.
“We are at a genuinely exciting moment in health AI. There’s just an enormous potential for everyone – patients, clinicians, and health systems–to benefit from these capabilities,” notes Pencina. “But,” he adds, “we need to make sure that everyone gets to share in those benefits, and the key to doing so is to ensure that the tools we create deliver meaningful improvements for patient care.”